Hard to Forget: Poisoning Attacks on Certified Machine Unlearning by Scott Alfeld, Cranium Advisor
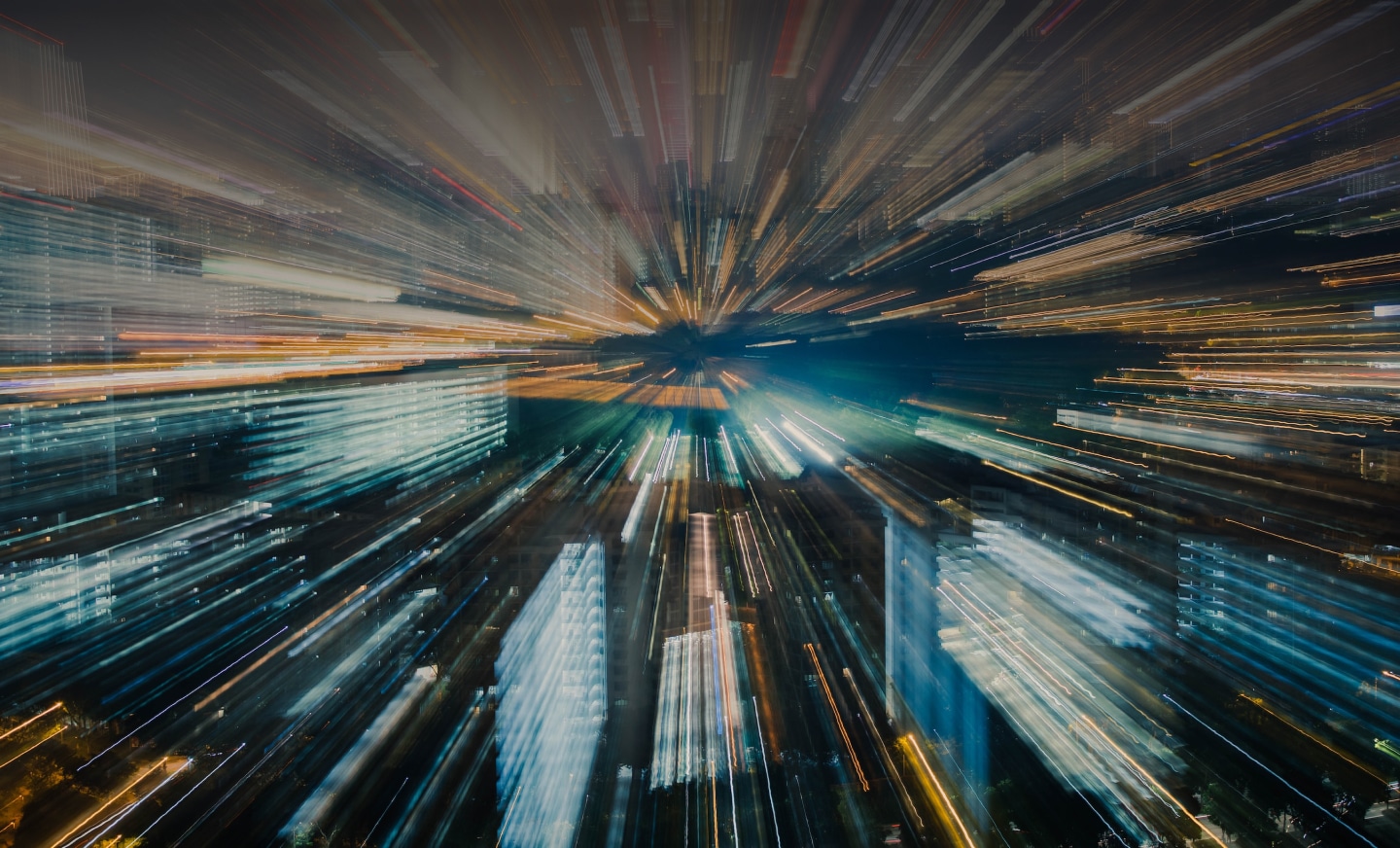
The right to erasure requires removal of a user’s information from data held by organizations, with rigorous interpretations extending to downstream products such as learned models. Retraining from scratch with the particular user’s data omitted fully removes its influence on the resulting model, but comes with a high computational cost.
Machine “unlearning” mitigates the cost incurred by full retraining: instead, models are updated incrementally, possibly only requiring retraining when approximation errors accumulate. Rapid progress has been made towards privacy guarantees on the indistinguishability of unlearned and retrained models, but current formalisms do not place practical bounds on computation.
In this paper we demonstrate how an attacker can exploit this oversight, highlighting a novel attack surface introduced by machine unlearning. We consider an attacker aiming to increase the computational cost of data removal. We derive and empirically investigate a poisoning attack on certified machine unlearning where strategically designed training data triggers complete retraining when removed.
Download the full paper here
Source:
Marchant, N. G., Rubinstein, B. I. P., & Alfeld, S. (2022). Hard to Forget: Poisoning Attacks on Certified Machine Unlearning. Proceedings of the AAAI Conference on Artificial Intelligence, 36(7), 7691-7700 https://doi.org/10.1609/aaai.v36i7.20736